Written by Jasper Lincoln '25
Edited by Lisa Liong '25
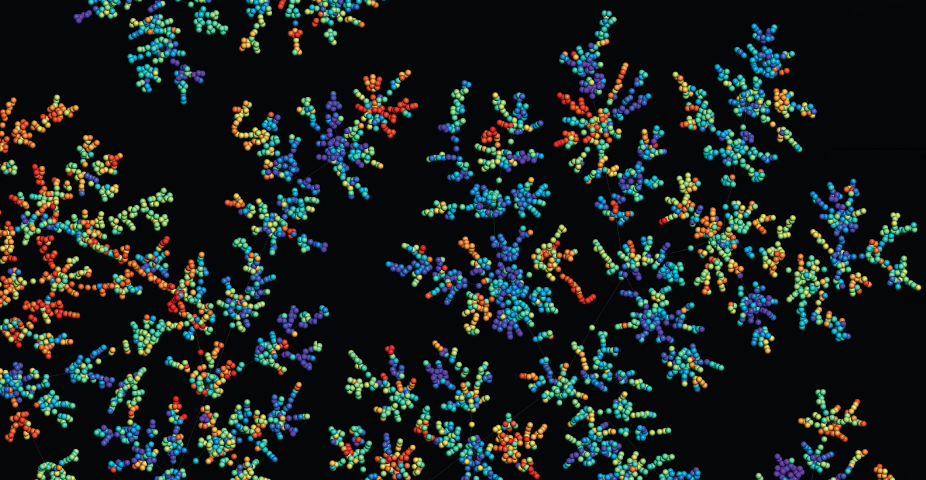
Image from C&EN [3]
Like outer space is explored by astronauts, astronomers, and astrophysicists, the chemical space, or the universe of chemicals that are possible to synthesize, is explored and navigated by chemists. From diving into the depths of the ocean to find new medicinal molecules in sea sponges to the decades of research dedicated to synthesize a compound, exploring the chemical space is an intrinsically tricky business
Since the dawn of chemistry, chemists have required trial and error to explore all possible compounds. With time, the development of theory in the 1800s and 1900s allowed researchers to predict what will happen in a test tube before it’s even tested, and how a reaction can be improved before it’s improved. However, these theories aren’t perfect, they still require much trial and error, and researchers need to spend years to garner a strong enough chemical intuition to be able to solve for example, syntheses for pharmaceuticals, and even then the rate of chemical research often takes years from start to design and finish, with much guessing and checking in between. But recently that has been changing. Researchers all over the world are working on software and robotics to fundamentally understand and carry out organic chemical reactions through intelligent automation.
Computers and algorithms have only been powerful enough to be useful for chemistry since the 1980s. The earliest appearance of computer science in chemistry research was entirely physics based. In these calculations, algorithms approximated quantum mechanics to guess precise properties for molecules such as the electronic energy levels of the molecule. But these calculations were limited: they were slow and were not accurate.
With the advance in artificial intelligence, researchers have started using deep learning algorithms to accelerate and replace these physics based chemistry calculations, and have taken the field of computational chemistry in entirely new directions.
Researchers at IBM have trained neural networks to learn the language of organic chemistry in order to map organic reactions, and to map out this “chemical space”. IUsing this notion of organic chemistry as a language, they quite literally used language processing algorithms (such as used in Siri) to group reactions into clusters and organize them into a “family tree” of reactions using a type of neural network called “attention based neural networks” [1]. These neural networks are used in language processing to selectively amplify or suppress parts of sentences and words to extract the most relevant information from the sentence. Just like language processing, these neural networks are used with the added benefit over other strategies by evaluating which specific parts of a reagent and reaction mechanism are most important . You can check out a family tree of reactions here, and even use their related artificial intelligence tool that will solve how to synthesize complex molecules. Below is a generated synthesis of Neotame.
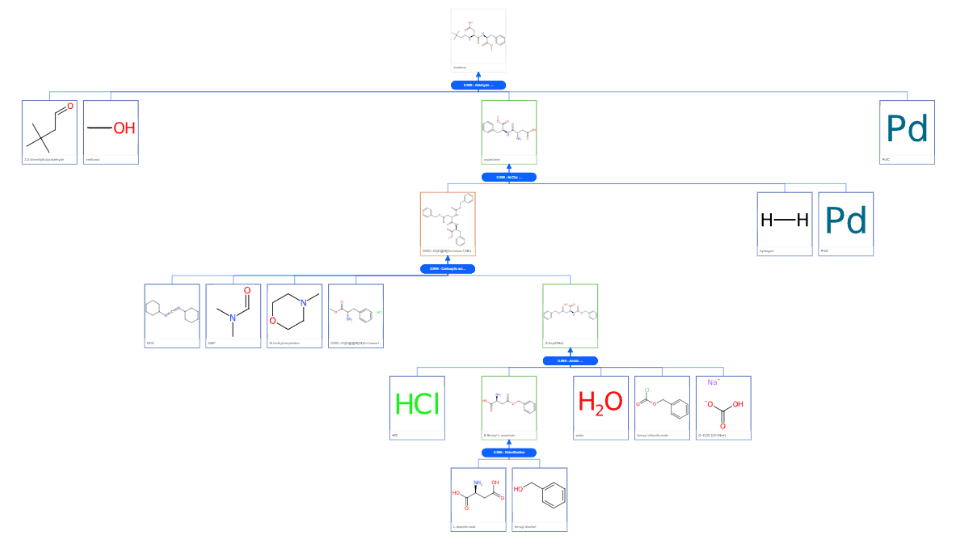
No matter what, strictly theoretical research has limitations in accuracy. The best way to model a system is in the system itself, so most things need to be tested in the real world at least once.
Researchers at the University of Glasgow, Scotland, led by Leroy Cronin, Jaraslow Granda, and Piotr Gromski, have taken robotic, machine learning, and AI into the laboratory.
Dubbed the “Chemputer,” it is not just a robot that reacts, separates, refines and analyzes compounds without human intervention, but it is an autonomous researcher of its own. Once the the Chemputer carries out a reaction, it takes raw data it has collected, such as spectrochemical data, yield, and molecular weight—all data that helps describe the product and compare it with its objective—and then it uses this data do create an even better procedure from before.
In essence they’ve overlapped the digitized language interpretation of chemical synthesis with robotic hardware and artificial intelligence that mirrors a human researcher.
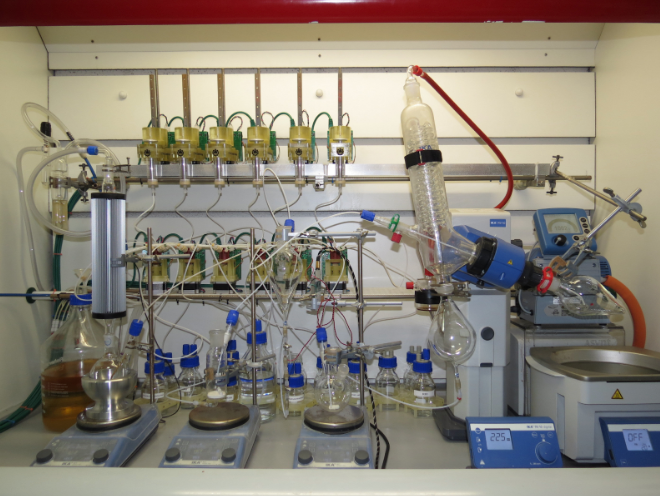
On top of the optimization and data analyzing machine learning used in the Chemputer, Cronin, Granda, and Gromsk have created a “curiosity” algorithm that mirrors the “curiosity driven learning” our brain carries out to maximize the frontiers of its knowledge.
The Chemputer is much more than a replacement for the tedious work a chemistry graduate student nowadays must do, it records and executes its work in a replicated manner and is able to carry out procedures much faster.
This is important. When automation is brought to any field, it expands its impact by orders of magnitude and in turn restructures what's possible in our society. With these developments, artificial intelligence will explore where humans alone have never explored before. We may see in the next few decades laboratory chemistry and biology become more automated even in the preliminary steps of research. It will be human researchers and AI, together exploring the chemical space.
References
[1] IBM RXN: New AI model boosts mapping of chemical reactions. IBM Research Blog 2021. https://research.ibm.com/blog/roborxn-designs-molecules (accessed April 15, 2023).
[2] Gromski PS, Granda JM, Cronin L. Universal Chemical Synthesis and Discovery with ‘The Chemputer.’ Trends in Chemistry 2020;2:4–12. https://doi.org/10.1016/j.trechm.2019.07.004.
[3] Exploring chemical space: Can AI take us where no human has gone before? n.d. https://cen.acs.org/physical-chemistry/computational-chemistry/Exploring-chemical-space-AI-take/98/i13 (accessed April 15, 2023).
Comments